Revolutionizing Household Chores: Insights from a Robotics Researcher
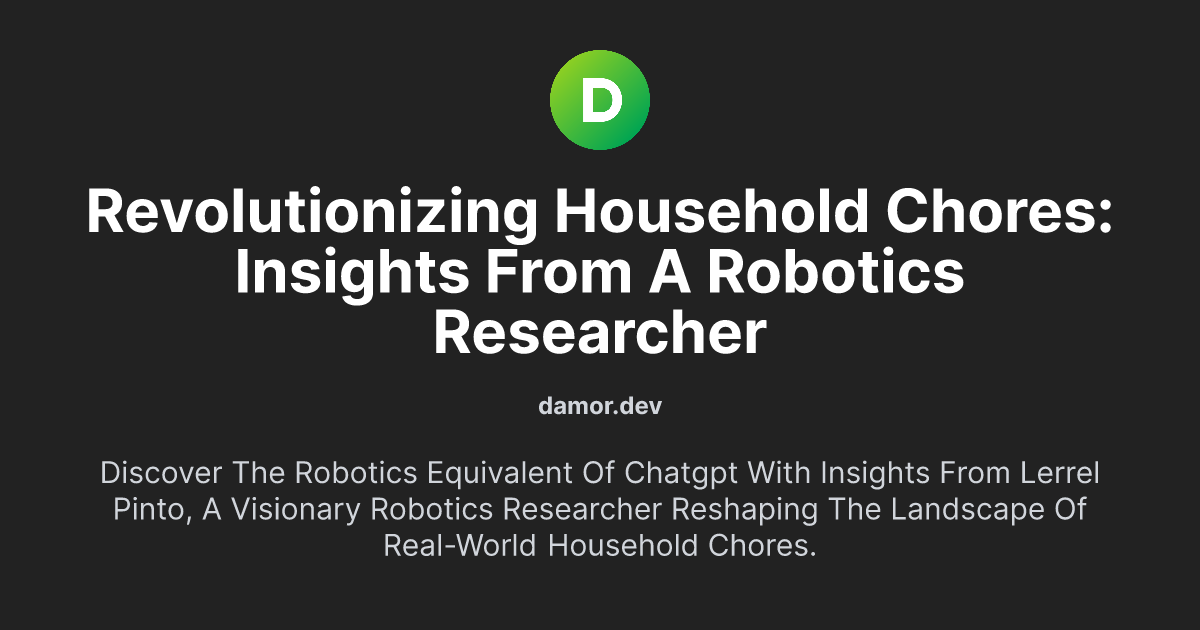
Building Real Robots for Household Chores
Lerrel Pinto, an assistant professor of computer science at NYU Courant, is making waves in the world of robotics and machine learning. His work, recognized on MIT Technology Review’s 2023 Innovators Under 35 list, focuses on solving real-world problems with robots.
Slow Progress in Robotics
Pinto acknowledges that progress in robotics is slower compared to other AI fields, but he sees it as a crucial challenge. In an interview, he emphasized the need to tackle real-world problems and move away from the trend of solving "toy problems" in lab environments.
Introducing Dobb-e: A Framework for Real-World Robot Learning
Pinto and his team recently introduced Dobb-e, an open-source framework designed to teach robots household manipulation skills. Unlike typical lab-based research, Dobb-e is aimed at addressing practical challenges faced in everyday homes.
Bridging the Gap with Generative AI
Pinto envisions robots seamlessly integrating into our daily lives, performing chores like doing dishes or laundry. He highlights the importance of generative AI, using data to teach robots various tasks. Despite the rapid progress in this field, Pinto notes challenges, including the bidirectional effect and the risk of bias in data.
Addressing Bias in Robotic Data
The issue of bias in robotic data is a significant concern. Since most data is generated by researchers, choices made during training impact where robots can effectively operate. Pinto gives an example of training a robot to open doors in affluent houses, causing issues when deployed in different environments.
Challenges Beyond Household Robotics
Pinto extends the discussion to self-driving cars, emphasizing the need for data collected in diverse environments. Mismatched data can lead to significant problems when deploying AI systems, highlighting the importance of addressing these challenges in various domains.
In summary, Lerrel Pinto's work aims to bring robots out of labs and into real-world scenarios, addressing challenges, biases, and practical applications in areas like household chores and beyond.