Meta's Breakthrough: Explore Emu Video & Emu Edit Generative AI Models
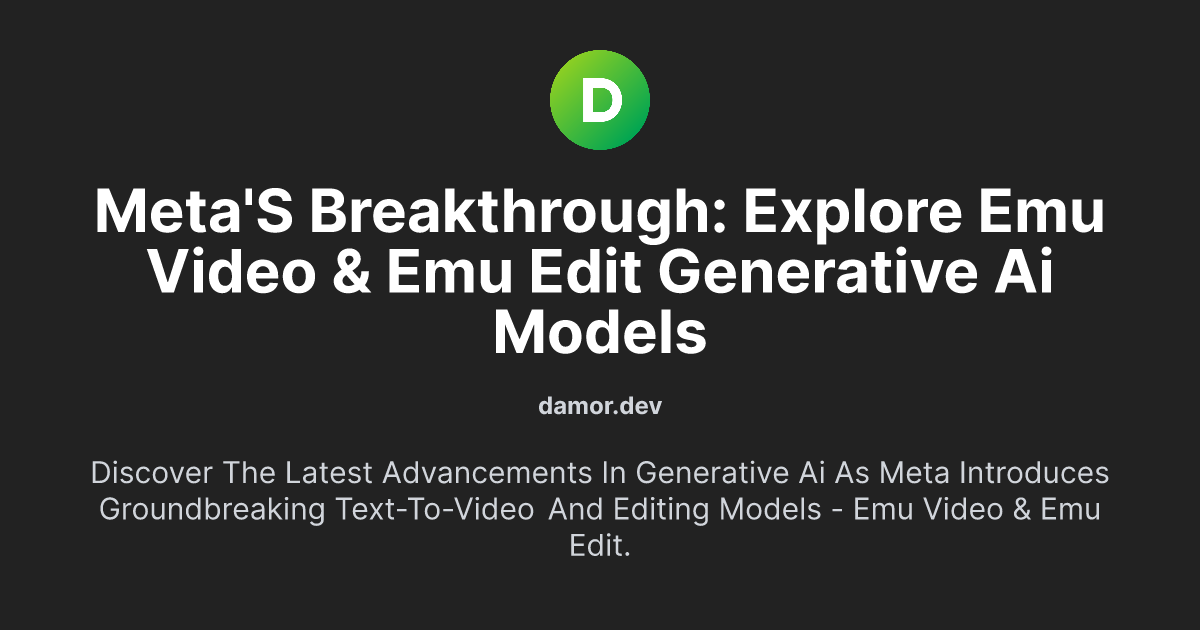
Meta Introduces New Generative AI Models: Emu Video & Emu Edit
Emu Video
Meta, led by Mark Zuckerberg, has made significant strides in generative AI with the introduction of two new models: Emu Video and Emu Edit.
Emu Video is a cutting-edge text-to-video generation model that follows a two-step process. First, it generates an image based on the provided text, and then it uses both the text and the generated image to create a high-quality, high-resolution video. The model achieves this by optimizing noise schedules for diffusion and employing multi-stage training.
Human evaluations show that Emu Video outperforms existing works, with preferences of 81% over Google’s Imagen Video, 90% over NVIDIA’s PYOCO, and an impressive 96% over Meta’s own Make-A-Video. It also surpasses commercial solutions like RunwayML’s Gen2 and Pika Labs. Notably, its approach is excellent for animating images based on user text prompts, outperforming previous works by 96%.
Emu Edit
Emu Edit is a versatile multi-task image editing model that excels in instruction-based image editing. It sets itself apart by outperforming existing models through training across various tasks, including region-based editing, free-form editing, and computer vision tasks.
The success of Emu Edit lies in its multi-task learning approach, utilizing learned task embeddings to accurately guide the generation process. The model showcases its versatility by generalizing to new tasks with minimal labeled examples, addressing scenarios with limited high-quality samples. It introduces a comprehensive benchmark with seven diverse image editing tasks for a thorough evaluation of instructable image editing models.
This model addresses the limitations of existing generative AI models in image editing by focusing on precise control and enhanced capabilities. It incorporates computer vision tasks as instructions, handling free-form editing tasks such as background manipulation, color transformations, and object detection. Unlike many existing models, Emu Edit precisely follows instructions, altering only the pixels relevant to the edit request.
Trained on a large dataset of 10 million synthesized samples, Emu Edit delivers unprecedented results in terms of instruction faithfulness and image quality. It establishes new state-of-the-art performance in both qualitative and quantitative evaluations for various image editing tasks.